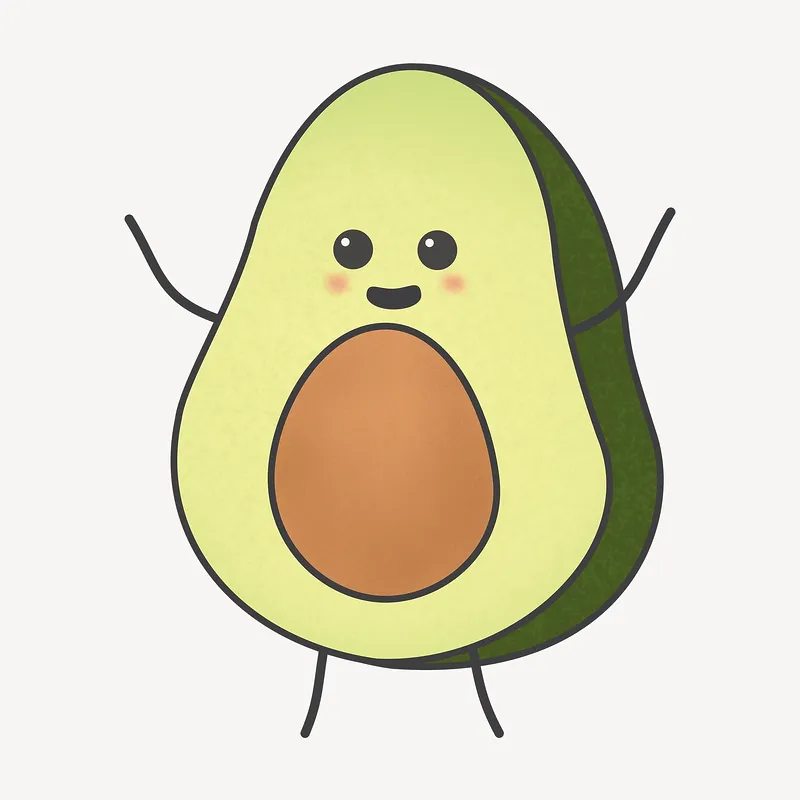
Huggingface Caching
Introduction Huggingface models can be quite large and require a lot of computational resources to train and run, which can be challenging for users who want to run these models on their local machines or in cloud-based environments. One solution to this problem is to use caching, which involves storing precomputed values so that they can be reused later without having to be recalculated. In the context of Hugging Face and transformer models, caching involves storing intermediate values that are generated during the processing of text data using a transformer model....